Understanding Statistical Significance in Research
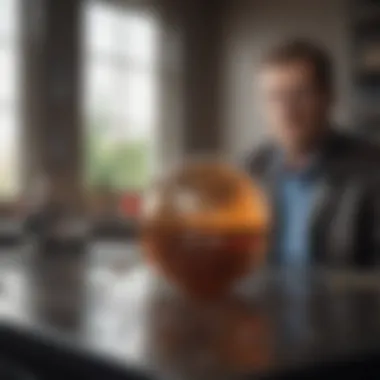

Intro
Statistical significance is a vital concept in research and data analysis. It helps determine if a result is likely due to chance or if it reflects a genuine effect. Understanding this concept is crucial for investors, researchers, and educators alike. When engaging with data, one needs to know not just the numbers, but also what they mean in context.
Why It Matters
Statistical significance plays a key role in decision-making processes across various fields. It informs whether the findings from a study are reliable or should be interpreted with caution. A common threshold used to determine this is the p-value. When the p-value is less than a certain threshold, typically 0.05, the result is often deemed statistically significant.
The implication of this is profound. For instance, in clinical trials, determining whether a new drug is effective requires understanding its statistical significance. If the results are significant, researchers can proceed with confidence. Conversely, non-significant results may lead to re-evaluation or additional studies.
Key Considerations
Before delving into calculating statistical significance, itβs essential to consider:
- Sample Size: A larger sample size can lead to more reliable results.
- Effect Size: This informs how substantial the effect is, not just if it is statistically significant.
- Confounding Variables: Understanding other factors that may influence the results is crucial.
"Statistical significance does not imply practical significance. Context is essential in interpreting results."
Throughout this guide, we will explore the foundational principles, methods of calculation, and the interpretation of results related to statistical significance. By the end, you will have a clearer understanding of how to apply these concepts to your own decision-making processes.
Understanding Statistical Significance
Statistical significance is a critical concept in research and data analysis. It determines whether the results obtained from a study are likely due to chance or if they reflect a true effect or relationship. Understanding this concept is essential for making informed decisions based on data.
Calculating statistical significance involves several key elements. The first is the formulation of hypotheses, which are predictions or assertions that will be tested through statistical methods. Inaccurate interpretation of statistical significance can lead to poor conclusions, which may affect entire fields of study, business investments, and policy decisions. By grasping the nuances of statistical significance, researchers and analysts can assess the reliability and validity of their findings.
Additionally, knowing how to calculate and interpret statistical significance empowers investors and decision-makers. For them, determining the significance of data can influence investment strategies and resource allocation. Awareness of this concept allows for more robust analysis of trends, leading to more sound financial decisions.
In this article section, we will delve deeper into foundational aspects of statistical significance, focusing on definitions, roles of statistical tests, and the relationships between various hypotheses.
Defining Statistical Significance
Statistical significance indicates the likelihood that a research result is not due to chance. It is often measured through p-values, which quantify the probability that the observed data would occur if the null hypothesis were true. Typically, a p-value less than 0.05 is considered statistically significant, suggesting a less than 5% likelihood that the results are due to random chance.
The concept is not merely about achieving a threshold; it is about understanding what that threshold represents. Statistical significance reflects the strength of the evidence against the null hypothesis. Therefore, itβs a precise measure that helps scholars, researchers, and professionals determine whether their findings have practical relevance.
The Role of Statistical Tests
Statistical tests serve as tools for researchers to evaluate their hypotheses. They systematically analyze data to determine whether the null hypothesis should be rejected in favor of the alternative hypothesis. Each test has its specific assumptions and application context.
For instance, the t-test compares the means of two groups, while ANOVA assesses multiple groups simultaneously. Choosing the appropriate test is crucial as it affects the validity of the conclusions drawn. Additionally, understanding these tests promotes better communication between researchers and stakeholders, allowing for more informed decisions based on statistical findings.
Statistical tests also help to ensure that results are robust. When researchers use these tests correctly, they can more confidently assert that their results reflect true effects rather than mere coincidence.
Null Hypothesis and Alternative Hypothesis
At the core of statistical significance lies the null hypothesis and its counterpart, the alternative hypothesis. The null hypothesis posits that there is no effect or relationship between variables, acting as a default assumption. Conversely, the alternative hypothesis suggests that a significant effect or relationship does exist.
Formulating these hypotheses is essential before conducting statistical tests. The testing process aims to provide evidence concerning the null hypothesis. If the data supports rejecting the null hypothesis, researchers can accept the alternative hypothesis with a certain level of confidence.
The relationship between these hypotheses is fundamental to understanding statistical significance. A well-constructed study begins with clear hypotheses, guiding the research process and ensuring meaningful interpretation of results.
"Statistical significance is not just a number; it is a representation of the evidence we have against a default assumption."
In summary, grasping the essence of statistical significance, along with the roles of hypotheses and tests, enhances the analytical proficiency of both new and experienced researchers and investors. This foundational knowledge lays the groundwork for the more complex calculations and interpretations that will follow in this guide.
Key Concepts in Statistical Analysis
The realm of statistical analysis is foundational in understanding data and making informed decisions based on empirical evidence. Emphasizing the importance of key concepts within this field allows researchers, investors, and analysts to navigate complexities inherent in data interpretation. The concepts covered, including P-values, confidence levels, and the power of statistical tests, deepen our comprehension of how statistical significance affects outcomes in various domains of study. Each element contributes uniquely to the broader picture, ensuring rigorous analysis in research settings.
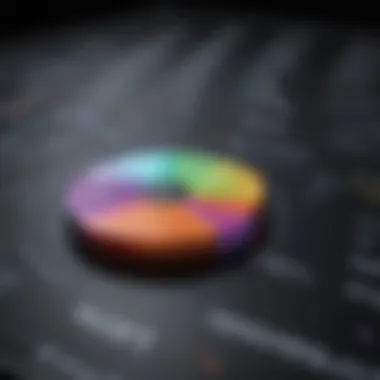

P-Values Explained
The P-value represents the probability that the observed results would occur under the assumption that the null hypothesis is true. This concept is pivotal because it aids in determining the strength of evidence against the null hypothesis. Generally, a smaller P-value indicates stronger evidence against the null hypothesis. A common threshold is 0.05; if the P-value is less than this, researchers often reject the null hypothesis. However, it's crucial to note that P-values do not convey the size or importance of an effect. Researchers should avoid interpreting the P-value in isolation, as it is influenced by the sample size and study design.
Confidence Levels and Intervals
Confidence levels and intervals provide vital information regarding the reliability of statistical estimates. A confidence level, expressed as a percentage, illustrates how certain one can be about the probability that the population parameter lies within a defined range. Commonly used levels include 90%, 95%, and 99%. The corresponding confidence interval gives a range of values, calculated from the sample data, that is likely to contain the true population parameter. Understanding these intervals helps quantify uncertainty and allows one to make more informed decisions. Intervals that are narrower indicate more precision, while wider intervals suggest more uncertainty in the estimate.
Power of a Statistical Test
The power of a statistical test refers to the probability that the test will correctly reject a false null hypothesis. High power is desirable because it increases the likelihood of detecting an effect when one exists. Power is influenced by several factors, including sample size, effect size, and significance level. Researchers strive for a power of at least 0.80, meaning there is an 80% chance of correctly rejecting a false null hypothesis. A well-powered study reduces the risk of Type II errors, where a true effect is missed, thus augmenting the credibility of the research findings.
Understanding these key concepts in statistical analysis is essential for rigorous research and accurate data interpretation.
Common Statistical Tests
Common statistical tests serve as essential tools in understanding and analyzing data. They allow researchers and analysts to draw conclusions about populations based on sample data. The results from these tests help in making decisions in various fields, including healthcare, business, and social sciences. Each statistical test is designed for specific data types and research questions, making it crucial to choose the correct one.
When selecting a test, consideration of the data type, distribution, sample size, and hypothesis is important. The choice significantly influences the validity of the conclusions drawn. Failure to select an appropriate test may lead to incorrect interpretations and decision-making, which can have serious implications.
T-Tests
T-tests are widely used to compare the means between two groups. The primary types of t-tests include independent t-tests, dependent t-tests, and one-sample t-tests. Each serves a distinct purpose. For instance, an independent t-test evaluates two separate groups while a dependent t-test assesses data from the same group at different times.
T-tests assume that the data are normally distributed and have homogeneity of variances. They provide a p-value to determine if there is a statistically significant difference between the means. This simple yet powerful tool is valuable when data collection occurs under controlled conditions.
Chi-Squared Tests
Chi-squared tests are utilized to examine the relationship between categorical variables. This test is particularly beneficial when dealing with frequency data. For example, a researcher might want to determine if there is an association between gender and voting preference.
Chi-squared tests generate a statistic that compares the observed frequencies to the expected frequencies. If the observed rates differ significantly from the expected rates, it indicates potential associations between the groups. Notably, the sample size must be adequate to ensure reliability in the results.
ANOVA (Analysis of Variance)
ANOVA is employed when comparing the means across three or more groups. It assesses variations within and between groups to determine if at least one group mean differs from the others. There are different types of ANOVA, including one-way and two-way, depending on the number of independent variables examined.
The outcome of ANOVA includes an F-statistic and a p-value. A significant p-value indicates the necessity for further analysis, typically via post-hoc tests, to ascertain which specific group means are different. ANOVA is crucial for experiments involving multiple groups, providing a comprehensive view of the data.
Regression Analysis
Regression analysis investigates the relationship between one dependent variable and one or more independent variables. This test helps in predicting outcomes and understanding relationships within the data. The most common types include linear regression and multiple regression.
In simple linear regression, the focus is on one independent variable predicting a dependent variable. On the other hand, multiple regression accounts for two or more independent variables. The outputs include coefficients that indicate the strength and direction of relationships, as well as an R-squared value that demonstrates the percentage of variance in the dependent variable explained by the independent variables. Regression analysis is a foundational technique in statistical modeling and forecasting.
Understanding these common tests is vital for any data-driven decision-making process. Choosing the right method ensures that the interpretations are robust and reliable.
Calculating Statistical Significance
Understanding how to calculate statistical significance is crucial in today's data-driven world. Statistical significance helps us determine if our results are due to chance or if they offer meaningful insights. This is not simply a matter of crunching numbers but involves critical thinking in interpreting data. Whether in finance, medicine, or social sciences, knowing when findings can be considered statistically significant can have far-reaching implications.
The process reveals patterns that inform decisions and bolster arguments in research. Correctly calculating statistical significance enhances the credibility of studies, fostering trust and validation in published results. Hence, neglecting the details of this process may lead to misguided conclusions, potentially impacting real-world applications negatively.
Step-by-Step Calculation Process
Calculating statistical significance involves several steps. First, one must clearly define the null hypothesis and the alternative hypothesis. The null hypothesis generally states that there is no effect or difference, while the alternative suggests otherwise. This distinction provides a firm basis for analysis.
Next, you select an appropriate statistical test based on the nature of your data and the research question. Common tests include T-tests for comparing means, Chi-squared tests for categorical data, and ANOVA for more than two groups.
After selecting a test, collect your data accurately and ensure it meets the test assumptions. Once this is done, perform the calculations, which usually involve obtaining a p-value. The p-value indicates the probability of observing your data, or something more extreme, assuming the null hypothesis is true.
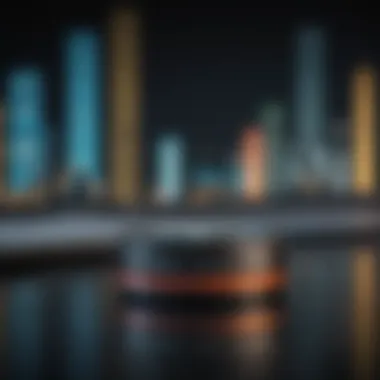
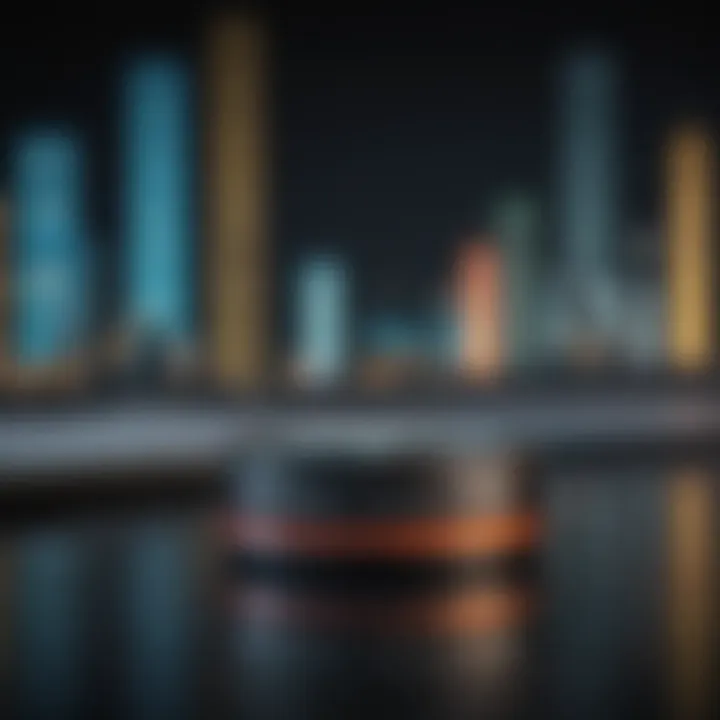
Finally, compare the p-value to your chosen significance level, typically set at 0.05. If the p-value is less than this threshold, you reject the null hypothesis, concluding that there is a statistically significant effect.
Using Software for Calculations
With the advancement of technology, statistical software has become indispensable in calculating statistical significance. Software like R, SPSS, and Python libraries streamline the process. These tools automate complex calculations, drastically reducing the potential for human error.
Many programs offer user-friendly interfaces that allow users to input data and specify the type of analysis required. This features significantly enhance efficiency, especially for large datasets. Additionally, results generated can provide comprehensive outputs, including confidence intervals and effect sizes, adding further context to your findings.
Manual Calculation vs. Software Tools
While software offers convenience, understanding manual calculations is also important. Manual calculations foster a deeper comprehension of the underlying mathematical concepts. Knowing how to compute p-values or confidence intervals by hand can empower researchers to identify errors or anomalies in software-generated results.
However, manual methods can be time-consuming and prone to error, particularly with large data sets. Therefore, a balanced approach is advisable. Beginners can benefit from learning both methods, while seasoned researchers often rely on software but engage in manual checks for accuracy and verification of results.
Interpreting Results and Implications
Interpreting results in statistical significance is crucial for any analysis. This section aims to shed light on how researchers and analysts can draw meaningful conclusions from their findings. Statistical significance provides a gauge for understanding whether observed patterns in data are genuine or mere flukes. When interpreting results, it is essential to look beyond simple p-values and consider broader implications.
Understanding P-Value Thresholds
P-values serve as a cornerstone in statistical testing. They indicate the probability of obtaining results at least as extreme as those observed, given that the null hypothesis is true. A common threshold for significance is 0.05. This means results with a p-value below 0.05 are often deemed statistically significant. However, relying strongly on this cutoff has its challenges.
- The choice of p-value threshold can vary based on the field and specific research context.
- Misinterpretation of p-values can lead to false conclusions. A very low p-value might seem meaningful but does not always imply a strong practical effect.
- Researchers need to consider the context of their study. For instance, biological or social sciences may require more stringent thresholds than fields like marketing.
Understanding these nuances in p-value thresholds can minimize misconceptions in research findings.
Real-World Applications of Statistical Significance
In practice, understanding statistical significance helps inform real-world decisions across various domains. Here are some applications:
- Healthcare: Statistical testing can help determine the effectiveness of a new medical treatment. Significance indicates whether an observed improvement in patient outcomes is likely due to the treatment rather than chance.
- Business: Companies rely on statistical significance to analyze consumer behavior and measure campaign effectiveness. A statistically significant increase in sales, for example, can warrant further investments.
- Public Policy: Policy makers use statistical significance to assess the impact of new regulations or programs. If a policy results in statistical significance in terms of improved outcomes, it may lead to broader implementation.
"Understanding the practical implications of statistical significance is vital for making informed and effective decisions."
Limitations of Statistical Significance
While the concept of statistical significance is valuable, it is not without limitations. Some critical points to consider include:
- Reliance solely on p-values may overshadow other important factors, such as effect size or practical significance.
- Statistical significance does not imply causation. It merely highlights an association, which could be coincidental or influenced by confounding variables.
- Publication bias can affect the body of research. Studies with non-significant results may go unpublished, leading to an inflated perception of significant findings in literature.
In summary, interpreting results and their implications is an intricate process. It extends beyond understanding p-values to incorporate real-world applications and recognizing the limitations inherent in statistical analyses.
The Debate Over Statistical Significance
The discussion surrounding statistical significance has evolved significantly in recent years. This debate touches upon various aspects of statistical analysis, influencing how researchers interpret their results and make decisions in fields ranging from medicine to social sciences. Understanding this debate is essential as it helps to clarify both the applications and limitations of statistical methods. It encourages a more nuanced approach to research, avoiding simplistic conclusions based solely on p-values. Below, we will explore two main areas of this debate: critiques of p-value use and alternatives to traditional statistical significance.
Critiques of P-Value Use
Criticism of p-values has intensified, raising important questions about their role in research. Many scholars argue that p-values can be misleading. A p-value, often set at a threshold of 0.05, indicates whether results are statistically significant but does not measure effect size or practical significance. This can lead to situations where results are reported as significant without considering their real-world implications.
Furthermore, the misuse of p-values can lead to a phenomenon known as the garden of forking paths. Here, researchers may unconsciously engage in multiple testing or selectively report results to achieve a statistically significant outcome. This can distort scientific evidence and create a biased view of results.
"Statistical significance does not imply practical importance. Researchers must consider the context and real-world implications of their findings."
Some experts argue that the binary nature of p-values, categorized as significant or not, oversimplifies complex data. This has sparked discussions on the need for a more comprehensive evaluation of evidence in research.
Alternatives to Statistical Significance
In light of the critiques regarding p-values, researchers have begun to explore several alternatives for interpreting data. Some of these alternatives include:
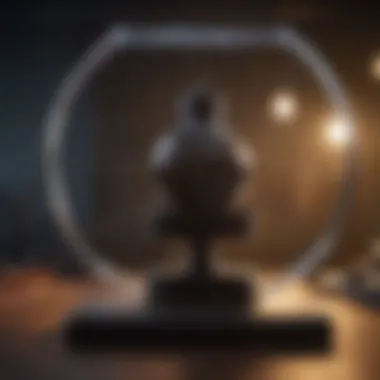
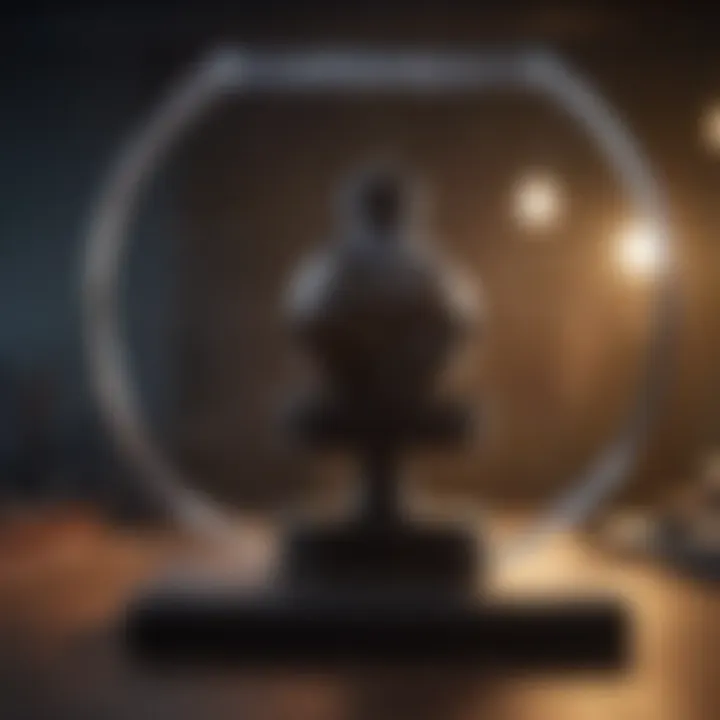
- Confidence Intervals: Instead of focusing on a single p-value, confidence intervals provide a range of values that are likely to contain the true effect size. This acknowledges uncertainty and offers more information about the precision of an estimate.
- Bayesian Methods: Bayesian statistics allows for incorporating prior knowledge into the analysis. This approach provides a framework for updating beliefs based on evidence, offering a more continuous interpretation of results compared to the dichotomy of p-values.
- Effect Sizes: Reporting effect sizes helps to convey the magnitude of a phenomenon rather than simply indicating whether it is present or not. This adds clarity and aids in understanding the practical significance of research findings.
These alternatives encourage researchers to approach data with a critical eye, considering both statistical and practical significance in their findings. They also promote transparency and rigor in research practices.
In summary, the debate over statistical significance highlights the need for careful interpretation and consideration of limits. As the dialogue in statistics continues to evolve, so should our approaches to analyzing and communicating research findings.
Practical Considerations in Research
In research, acknowledging the practical aspects of statistical significance is crucial. These considerations influence the design, implementation, and interpretation of studies. Each element shapes the outcomes and how researchers communicate their findings. This section will explore the key areas of focus when dealing with statistical significance: appropriate test selection, determining sample size, and the ethical ramifications.
Choosing the Right Test
Selecting the right statistical test is one of the foundational steps in the research process. The choice is determined by the type of data being analyzed and the research question. For instance, if the data is normally distributed, parametric tests like the t-test or ANOVA might be appropriate. However, if the data does not meet these assumptions, non-parametric tests, like the Mann-Whitney U test, become necessary. Misapplying tests can lead to inaccurate conclusions.
It is also essential to consider the number of groups being compared and the nature of the variables involved. Are you working with categorical data? Chi-squared tests would be the best fit. Alternatively, if assessing relationships between variables, regression analysis might be suitable. Understanding the assumptions behind each test helps ensure valid results and affects statistical significance profoundly.
Sample Size and Its Impact
The sample size plays a crucial role in statistical analysis. Larger sample sizes generally lead to more reliable results. When a sample is too small, it can result in a lack of power to detect true effects of a treatment or intervention. This issue can produce misleading outcomes.
Researchers should conduct power analysis before beginning a study. This assists in determining the minimum sample size needed to detect an effect of a certain size with a desired level of significance. A well-calibrated sample size not only enhances the validity of the findings but also contributes to ethical research practices by ensuring that resources are utilized efficiently.
Ethical Implications of Significance Testing
Ethics in research cannot be overlooked, especially concerning significance testing. The potential for misuse of statistical results raises serious concerns. For example, p-hacking can lead to misleading interpretations, where researchers may try various tests and only report those that yield significant results. This practice undermines credibility and can lead to a wider mistrust of research findings.
Additionally, the communication of results must be handled ethically. It is essential to report statistical significance and effect sizes transparently. Overstating findings can mislead stakeholders and influence decision-making inappropriately. Researchers must strive to maintain integrity in reporting, ensuring they present a balanced view of their findings.
"Transparency and ethics in statistical reporting foster trust and promote sound decision-making in research and beyond."
By emphasizing these practical considerations, researchers can minimize potential pitfalls. Understanding tests, ensuring adequate sample sizes, and adhering to ethical practices are significant for responsible research. This approach ultimately enhances the credibility and usability of statistical significance in real-world applications.
Resources for Further Learning
In the realm of statistical significance, having access to quality resources is crucial for both learning and applying the concepts effectively. This section highlights the importance of engaging with diverse educational materials that can enhance understanding and proficiency in statistical methods. The process of learning is not only about absorbing information but also about developing the skills necessary to apply that knowledge in real-world situations. By utilizing various resources, individuals can tailor their learning experience to suit their own unique needs and pace.
Books on Statistical Methods
Books are a foundational resource for learning statistical methods. They provide structured knowledge and a deep dive into various topics. Some renowned books include "The Elements of Statistical Learning" and "Statistics" by David Freedman. These texts often explore fundamental concepts and real-life applications, making them valuable for both beginners and seasoned practitioners.
When selecting books, consider the following points:
- Depth of Content: Look for books that detail both theory and application.
- Author Expertise: Authors with solid backgrounds in statistics can provide invaluable insights.
- User Reviews: Check reviews to gauge the book's effectiveness and clarity.
Many of these books are also available in digital formats, allowing for easy access and portability.
Online Courses and Tutorials
The internet has transformed education, making it more accessible. Online courses on platforms like Coursera and edX offer structured learning environments with flexibility. These courses often feature video lectures, quizzes, and peer interactions, enhancing the experience. It is advisable to select courses that include hands-on projects since practical application is essential in learning statistics.
A few platforms to consider are:
- Coursera: Offers courses from leading universities.
- edX: Has many options across various levels of complexity.
- Khan Academy: Provides free tutorials on basic to advanced statistical concepts.
Explore different formats, such as webinars and video tutorials, to find what suits your learning style best.
Statistical Software Documentation
As statistical analysis increasingly relies on software tools, understanding these applications is imperative. Good documentation helps users navigate software features and functionalities. Programs like R, SPSS, and Python libraries provide comprehensive manuals and guides.
Key elements to consider when consulting software documentation include:
- User-Friendliness: Good documentation should be easy to navigate.
- Examples and Tutorials: Practical examples facilitate better understanding.
- Community Forums: Engaging with communities can provide additional support and insights.
Known resources like Wikipedia can serve as entry points, linking to specific documentation and user forums.
In summary, leveraging books, online courses, and software documentation will significantly benefit anyone looking to improve their understanding of statistical significance. Incorporating these resources into your learning journey creates a robust foundation for interpreting data and making informed decisions.